Have you ever wondered how your smartphone camera can detect your smiling face and capture the perfect selfie? Or how your car’s navigation system can understand your frustration when you get stuck in traffic?
The answer lies in emotion recognition technology. This cutting-edge technology enables machines to identify and interpret human emotions through various techniques. They may include facial recognition, speech recognition, and physiological signals.
In this article, we’ll dive deeper into emotion recognition technology, exploring its definition and potential use cases.
Table of Contents
What Is Emotion Recognition Technology?
Emotion recognition technology is a branch of artificial intelligence (AI) that enables machines to identify, interpret, and respond to human emotions. This technology utilizes various techniques, including machine learning algorithms, datasets, and training data, to analyze facial emotions, vocal tones, and physiological signals such as heart rate and skin conductance.
Facial emotion recognition is one of the most common techniques used in emotion recognition technology. It involves analyzing facial expressions such as smiles, frowns, and eyebrow movements to determine a person’s emotional state. This technique is commonly used in customer service, where companies monitor customer satisfaction levels and provide personalized services based on their emotions.
Sentiment analysis is another popular application of emotion recognition technology, which involves analyzing text and speech to determine the writer’s or speaker’s emotional state. This technique is often used in social media analysis and market research, where companies use it to gauge customer opinions and preferences.
Emotion Recognition Use Cases
Emotion recognition technology has a wide range of use cases across various industries. Here are some examples:
1. Self-driving vehicles
Emotion recognition technology can be used in self-driving cars to ensure the safety of passengers. The technology can use in-car speech recognition to analyze passengers’ facial expressions and alert the vehicle’s system if the passenger appears tired or distracted.
2. Virtual assistants
Virtual assistants like Siri, Alexa, and Google Assistant use emotion recognition technology to understand users’ emotions and respond appropriately. For example, if a user sounds sad or frustrated, the virtual assistant may offer comforting words or suggest calming music.
3. Medical diagnostics
Emotion recognition technology can be used in medical diagnostics to help doctors and healthcare professionals identify and diagnose mental health disorders. The technology can analyze facial expressions, voice tones, and other physiological signals to identify patterns and potential health issues.
4. Smart retail
Retailers can use emotion recognition technology to analyze shoppers’ facial expressions and behaviors to understand their shopping preferences and provide personalized recommendations. For instance, a retailer can use this technology to determine which products shoppers are more likely to purchase based on their emotional response to specific products.
5. Personalized education
Emotion recognition technology can also be used in personalized education. For example, educational software can analyze students’ facial expressions and tone of voice to determine their level of engagement and understanding of the material. Based on this information, the software can adapt the lesson plan to meet student’s needs and provide more personalized instruction.
As technology continues to evolve and improve, we can expect to see even more innovative and exciting use cases in the future.
Challenges in Speech Emotion Recognition
Speech emotion recognition (SER) is a field of study within the emotion recognition technology model that focuses on identifying and interpreting emotions in human speech. While the technology has shown promising results, several challenges still need to be addressed.
Here are some of the main challenges in speech emotion recognition:
- Ambiguity in speech: The same words or phrases can be spoken with different emotions, making it challenging for SER systems to recognize emotions accurately. Additionally, people may use sarcasm or irony in their speech, which can be difficult to detect.
- Variability in speech: Emotions can be conveyed through various aspects of speech, such as pitch, tone, and volume, but these aspects can vary significantly between speakers, making it challenging to develop a system that can recognize emotions across a broad range of speakers.
- Contextual variability: The meaning of a sentence or phrase can change depending on the context in which it is spoken. SER systems must recognize and account for these contextual variations to accurately identify emotions in speech.
- Limited training data: Developing accurate SER systems requires high-quality training data. However, obtaining such data can be challenging, particularly for underrepresented emotions or populations.
- Ethical considerations: As with any technology that involves the collection and analysis of personal data, SER raises ethical concerns around privacy, consent, and potential misuse.
Curating a Dataset
Curating a high-quality dataset is essential for developing accurate and reliable emotion recognition systems. To create such datasets, it is important to carefully consider factors such as the diversity of emotions and demographics represented in the data, the dataset’s size, and the data’s quality.
One way to create high-quality datasets is through data collection services. These services use various methods, such as crowdsourcing, social media scraping, and targeted sampling, to collect large amounts of data from diverse sources. They can also provide data labeling and annotation tools to ensure that the data is accurately labeled with the appropriate emotion categories.
Using data collection services can be particularly beneficial for smaller companies or research teams needing more resources or expertise to collect and label their datasets. By outsourcing the data collection process, these teams can save time and resources while ensuring they have access to high-quality data.
Conclusion
Emotion recognition technology is a powerful tool that can enhance our understanding of human emotions and help us create personalized experiences in various domains such as healthcare, education, and marketing.
This technology has vast potential to revolutionize the way we interact with machines and each other. However, it is important to consider this technology’s ethical implications, including privacy, consent, and potential bias.
As emotion recognition technology advances and becomes more widely used, we must address these concerns and ensure that its implementation is responsible and beneficial for all.
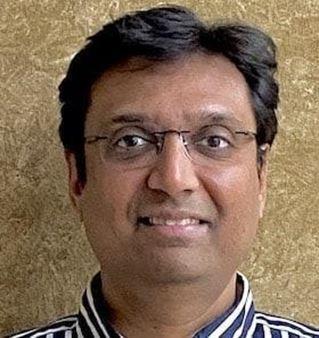
Vatsal Ghiya is a serial entrepreneur with more than 20 years of experience in healthcare AI software and services. He is the CEO and co-founder of Shaip, which enables the on-demand scaling of our platform, processes, and people for companies with the most demanding machine learning and artificial intelligence initiatives.